Biomedical Research
Examples of Current Projects
Prediction of Pulmonary and Cardiac Diseases using Echocardiograms
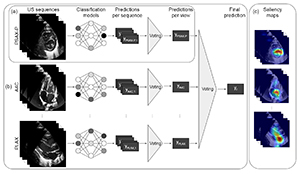
Pulmonary and cardiac diseases in newborns and younger children contribute to morbidity and mortality. Accurate and early detection of these diseases is crucial for a successful management. Using echocardiography, the primary diagnostic tool in paediatrics, human assessment is both time-consuming and expertise-demanding, raising the need for an automated and streamlined approach. In collaboration with the Hospital Barmherzige Brüder Regensburg, we are developing automated deep learning methods to assess these pulmonary and cardiac diseases. Since the applicability of deep learning methods is still limited in the medical domain due to their black-box nature our goal is to complement our predictions with additional model explanations such that the clinician can perform a sanity check before deciding whether to follow the model’s predictions or recommendations.
Predicting the Diagnosis, Management, and Severity of Paediatric Appendicitis from Ultrasound Images
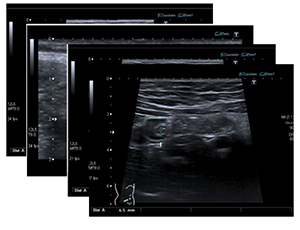
Appendicitis is a common childhood disease with the highest incidence rate between 10 and 19 years of age. Especially un-diagnosed acute appendicitis can cause ruptures and death in the worst case. To this day, there exists neither specific biomarkers for predicting acute appendicitis nor international guidelines for managing it. Currently, physicians rely on a combination of clinical, laboratory, and imaging data but misdiagnosis is common. In collaboration with the Children’s Hospital St. Hedwig in Regensburg, we work on interpretable neural network predictions to support physicians in diagnosing, assessing severity, and deciding the management of appendicitis using ultrasound images combined with clinical variables. Model interpretability is a central objective in our efforts since physicians are only willing to use machine learning tools if the model prediction can be explained.
StimuLOOP
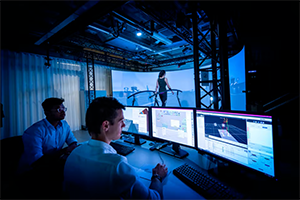
The StimuLOOP project investigates precision neurorehabilitation to improve gait of Stroke and Parkinson patients in a comprehensive study conducted by a group of scientists from ETH, UZH, USZ and the Cereneo Foundation. Conventionally, neurorehabilitation includes treatments to reduce disability, but their efficacies vary largely between individuals and frequently fail to achieve meaningful outcomes. The StimuLOOP project tries to address this issue by placing selected individuals into a personalized rehabilitation loop. While in this loop, gait quality of each individual is regularly measured, based on which feedback is given to the patients and gait adaptations are performed. The therapeutic process is then consolidated by performing sleep intervention to improve therapy sustainability while resting. The whole process is repeated almost daily over several weeks, leading to vast amounts of data of a multitude of modalities that are collected and provided to our group. Taking advantage of the amassed data, we aim to go beyond personalizing neurorehabilitation and apply state of the art machine learning methods to answer clinically relevant questions like: What are gait pattern correlations between Stroke and Parkinson Patients? Are there similarities in the rehabilitation learning process? Are initial measurements predictive of therapy success?
Prediction of Hypoglycemia in Children with Diabetes
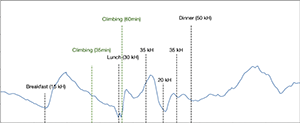
Hypoglycemia is the most common complication of type 1 diabetes. Previous studies found that nocturnal hypoglycemia is frequent in diabetic children and often remains unnoticed. An important risk factor for such hypoglycemic events is physical activity (PA) during the day. At the same time, however, regular engagement in sports or exercises is an important cornerstone for long-term diabetes treatment. Together with physicians from the University Children’s Hospital Basel (UKBB) we aim to leverage modern AI models to predict the effect of PA on the blood glucose level of patients to avoid hypoglycemic events. To allow for personalized predictions, we aim to integrate patient information about physical activity, insulin dosing, and nutrition, which has been collected in a controlled study by our collaborators from UKBB.